Diagnosing Skin Cancer using Machine Learning Techniques

DOI:
https://doi.org/10.54060/jase.2022.11Keywords:
Skin Cancer, Acid corrosion, MelanomaAbstract
Melanoma skin cancer is one of the deadly types of skin cancer and Biopsy is the method that is used to detect this cancer and success rate depends on performance of a trained doctor. The biopsy Process is very painful and requires considerable time. So, there is a need for a technique that can detect melanoma cancer that could avoid biopsy and that would be based on looking deep into skin cancer images. This paper has conducted a study on image classification of melanoma skin cancer using ma-chine learning and various neural network techniques. The stages of the cancer im-age classification process melanoma skin in this study include the preprocessing process, segmentation, feature extraction with ABCD namely Asymmetry, Border Irregularity, Color Variation and Diameter. Subsequently, it's quantified that both the machine learning and neural network can be used for skin cancer diagnostics.
Downloads
References
A. Baldi, P. Pasquali, and E. P. Spugnini, Skin Cancer: A Practical Approach. Springer Science & Business Media, 2013, ISBN 978-1-4614-7357-2
R. A. Schwartz and R. A. Schwartz, Introduction. In Skin Cancer. 2008. doi:10.1002/9780470696347
T. Wolff, E. Tai, and T. Miller, “Screening for skin cancer: an update of the evidence for the U.S. Preventive Services Task Force,” Ann. Intern. Med., vol. 150, no. 3, pp. 194–198, 2009. Introduction. Available from: https://www.ncbi.nlm.nih.gov/books/NBK34047/
T. Saida, Melanoma and Non-Melanoma Skin Cancers. Gan to kagaku ryoho. Cancer & chemotherapy. 2020.
P. Hoskin, “Skin cancer” 2020. 10.1201/9781315267081-19.
A. Krizhevsky, I. Sutskever, and G. E. Hinton, “ImageNet classification with deep convolutional neural networks,” Commun. ACM, vol. 60, no. 6, pp. 84–90, 2017.
M. P. Hendaria, S. Maliawan, U. Pusat, S. Denpasar, and K. S. Skuamosa, “Kanker kulit,” Kanker kulit. Kanker Kulit, pp. 1–17, 2013.
S. T. Tan, I. P. Dewi, B. Ilmu, and K. Kulit, “Melanoma Maligna”, vol. 42(12), pp. 908–913, 2015.
H. W. Higgins 2nd, K. C. Lee, A. Galan, and D. J. Leffell, “Melanoma in situ: Part I. Epidemiology, screening, and clinical features,” J. Am. Acad. Dermatol., vol. 73, no. 2, pp. 181–90, quiz 191–2, 2015.
E. Perera, N. Gnaneswaran, R. Jennens, & R. Sinclair, “Malignant Melanoma”, Healthcare, vol. 2(1), pp. 1–19, 2013.
H. W. Higgins 2nd, K. C. Lee, A. Galan, and D. J. Leffell, “Melanoma in situ: Part I. Epidemiology, screening, and clinical features,” J. Am. Acad. Dermatol., vol. 73, no. 2, pp. 181–90, quiz 191–2, 2015.
D. Purnamasari, J. Henharta, Y. P. Sasmita, F. Ihsani, and I. W. S. Wicaksana, Get Easy Using Weka. 2013.
S. Ben-David, Understanding Machine Learning: From Theory to Algorithms. 2014.
S. Barve, “Optical Character Recognition Using Artificial Neural Network,” International Journal of Advanced Research in Computer Engineering & Technology, vol. 1, no. 4, 2012., ISSN: 2278 – 1323
A. Karpathy, “Introduction to Convolutional Neural Networks”, 2018.
A. Esteva, B. Kuprel, R. A. Novoa, J. Ko, S. M. Swetter, H. M. Blau, & S. Thrun, “Dermatologist-level classification of skin cancer with deep neural networks”, Nature, vol. 542(7639), pp. 115–118, 2017.
R. B. Aswin, J. A. Jaleel, and S. Salim, “Hybrid genetic algorithm — Artificial neural network classifier for skin cancer detec-tion,” in 2014 International Conference on Control, Instrumentation, Communication and Computational Technologies (ICCICCT), 2014.
S. Mustafa, A. B. Dauda, and M. Dauda, “Image processing and SVM classification for melanoma detection,” in 2017 In-ternational Conference on Computing Networking and Informatics (ICCNI), 2017.
A. Esteva, B. Kuprel, R. A. Novoa, J. Ko, S. M. Swetter, H. M. Blau, & S. Thrun, (2017). “Dermatologist-level classification of skin cancer with deep neural networks”, Nature, vol. 542(7639), pp. 115–118, 2017.
E. V. Putten, A. Kambod, and M. Kambod, “Deep residual neural networks for automated Basal Cell Carcinoma detection,” in 2018 IEEE EMBS International Conference on Biomedical & Health Informatics (BHI), 2018.
A. Balakin et al., “Terahertz image processing for the skin cancer diagnostic,” in 2014 39th International Conference on Infrared, Millimeter, and Terahertz waves (IRMMW-THz), 2014.
A. Esteva, B. Kuprel, R. Novoa et al., “Dermatologist-level classification of skin cancer with deep neural networks”, Nature, vol. 542, pp. 115–118, 2017. https://doi.org/10.1038/nature21056
S. Mustafa, A. B. Dauda, and M. Dauda, “Image processing and SVM classification for melanoma detection,” in 2017 In-ternational Conference on Computing Networking and Informatics (ICCNI), 2017. 10.1109/ICCNI.2017.8123777.
E. V. Putten, A. Kambod, and M. Kambod, “Deep residual neural networks for automated Basal Cell Carcinoma detection,” in 2018 IEEE EMBS International Conference on Biomedical & Health Informatics (BHI), 2018. 10.1109/BHI.2018.8333437.
R. B. Aswin, J. A. Jaleel and S. Salim, “Hybrid genetic algorithm — Artificial neural network classifier for skin cancer detec-tion”, International Conference on Control, Instrumentation, Communication and Computational Technologies (ICCICCT), Kanyakumari, pp. 1304-1309, 2014. doi: 10.1109/ICCICCT.2014.6993162.
A. Krizhevsky, I. Sutskever, and G. E. Hinton, “ImageNet classification with deep convolutional neural networks,” Commun. ACM, vol. 60, no. 6, pp. 84–90, 2017.,https://papers.nips.cc/paper/4824-imagenet-classification-with-deep-convolutional-neural-networks.pdf
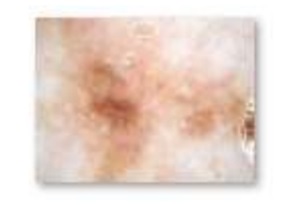
Downloads
Published
How to Cite
CITATION COUNT
Issue
Section
License
Copyright (c) 2022 Dr Anuradha Pillai

This work is licensed under a Creative Commons Attribution 4.0 International License.