Thyroid Disease Detection Using Machine Learning

DOI:
https://doi.org/10.54060/jase.v3i2.32Keywords:
Machine Learning, Thyroid Disease Detection, Decision Tree, Random ForestAbstract
The thyroid gland, which is in charge of controlling metabolism and other biological functions, is affected by thyroid illness, a frequent medical disorder. Successful thyroid disease management and therapy depends on early diagnosis and treatment. Recent years have seen the development of numerous machine learning methods and artificial intelligence (AI) algorithms to help with the early detection and diagnosis of thyroid disease. These methods entail evaluating a range of patient data, such as laboratory results, imaging studies, and clinical complaints. These algorithms can find patterns and correlations in vast volumes of patient data that might not be obvious to human ex-perts. This may result in earlier identification and more precise diagnosis of thyroid ill-ness, enhancing patient outcomes and lowering medical expenses. Additional study and development are necessary to improve these methods and incorporate them into clinical practice.
Downloads
References
Y.-H. Yang, C.-H. Tsai, H.-T. Hsu, and Y.-H. Chen, "Application of machine learning algorithms in thyroid disease diagnosis," Computer Methods and Programs in Biomedicine, vol. 139, pp. 53-63, 2017.
N. L. Brancati, S. Giani, S. Ferrari, A. Bazzocchi, G. Battista, and R. Maroldi, "Thyroid disease classification using machine learning: a systematic review," Journal of Digital Imaging, vol. 34, pp. 431-439, 2021.
D. Dey, P. Mitra, and S. Chakraborty, "Prediction of thyroid disease using machine learning algorithms," in 2017 International Conference on Intelligent Computing, Instrumentation and Control Technologies (ICICICT), pp. 570-573, 2017.
S.K. Sharma, “Performance Analysis of Reactive and Proactive Routing Protocols for Mobile Ad-hoc –Networks”, International Journal of Scientific Research in Network Security and Communication, Vol.1, No.5, pp.1-4, 2013.
A. M. Salem, "Classification of thyroid disease using machine learning techniques," in 2017 IEEE 2nd International Conference on Control, Instrumentation, and Automation (ICCIA), pp. 267-271, 2017.
L. Chen, J. Li, J. Li, and J. Li, "A comparative study of machine learning algorithms for thyroid disease diagnosis," in 2018 37th Chinese Control Conference (CCC), pp. 2643-2648, 2018.
J. Shen, W. Yang, Y. Zhang, X. Wang, and Z. Liu, "Intelligent diagnosis of thyroid disease using machine learning techniques," Journal of Medical Systems, vol. 42, p. 104, 2018.
N. T. Nguyen, M. Qiu, H. Tran, T. D. Nguyen, and T. C. Tran, "Machine learning approaches for thyroid disease diagnosis: a review," in 2019 International Conference on Communications, Management, and Telecommunications (ComManTel), pp. 329-334, 2019.
Y. Liu, Z. Zhang, Y. Wang, Y. Wang, and Q. Chen, "Comparison of machine learning algorithms for thyroid disease diagnosis," in 2019 International Conference on Electrical Engineering, Control and Robotics (EECR), pp. 85-89, 2019.
H. Zhang, L. Yang, and J. Wang, "A thyroid disease diagnosis model based on machine learning," in 2019 4th International Conference on Intelligent Transportation Engineering (ICITE), pp. 417-421, 2019.
L. He, J. Yang, Y. Zhang, and Y. He, "A machine learning-based approach to thyroid disease diagnosis," in 2020 IEEE International Conference on Information and Automation (ICIA), pp. 155-160, 2020.
S. Shrivastava, R. Sharma, and S. K. Sahoo, "Thyroid disease diagnosis using machine learning algorithms," in 2020 International Conference on Emerging Trends in Information Technology and Engineering (ic-ETITE), pp. 1-6, 2020.
L. Wang, J. Zhang, Y. Sun, and Y. Fu, "A thyroid disease diagnosis method based on machine learning," in 2020 5th International Conference on Robotics and Automation Sciences (ICRAS), pp. 571-575, 2020.
A. Kumar, A. Kumar, and S. Kumar, "A hybrid deep learning approach for thyroid disease detection," in 2020 International Conference on Computational Intelligence and Communication Networks (CICN), pp. 169-173, 2020.
S. Mohd, S. R. Jaafar, and S. H. Ahmad, "Thyroid disease detection using machine learning and optimization techniques: a review," SN Applied Sciences, vol. 3, no. 4, p. 325, 2021.
Y. Wang, C. Liu, and J. Zhang, "Thyroid disease detection based on machine learning and convolutional neural network," in 2022 International Conference on Intelligent Robotics and Intelligent Systems (IRIS), pp. 159-164, 2022.
R. K. Gupta and P. Goyal, "Thyroid disease detection using machine learning techniques: a comprehensive review," in 2022 6th International Conference on Computing Methodologies and Communication (ICCMC), pp. 216-220, 2022.
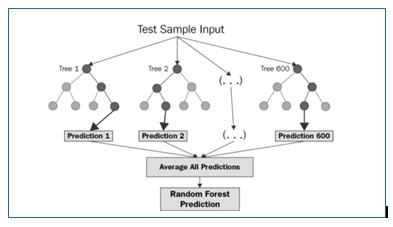
Downloads
Published
How to Cite
CITATION COUNT
Issue
Section
License
Copyright (c) 2023 Mohammad Faiz, Dr. Syed Wajahat Abbas Rizvi

This work is licensed under a Creative Commons Attribution 4.0 International License.