Role of Mathematics in Neuroscience

DOI:
https://doi.org/10.54060/jase.v3i2.16Keywords:
Neuroscience, Adsorption parameter, actualities, connectionAbstract
With the growing role and application of mathematics in almost every field it is no doubt that it plays an experimental and crucial role in an advancing field of neurosci-ence which is still under constant research. The aim of the report is two fundamental points: to show how mathematical models that enlighten a few pieces of neuroscience can be built, principally by depicting both "exemplary" and recent models; what's more, to make sense of mathematical techniques by which these models can be investigated, in this way yielding pre-expressions and clarifications that can be applied as a powerful influence for experimental information. To show how somewhat straightforward mathematical models and their investigations can help comprehension of certain areas and activity of brain and central nervous systems. In computational neuroscience a model is recorded which is an estimate of the connection between a bunch of infor-mation; however, there is no formal intelligent way to "demonstrate" the model right or wrong. So mathematical recreations are done. The role of mathematics in neuroscience has been elaborated and discussed.
Downloads
References
P. Eckhoff, P. Holmes, W. C. by Sadeghi, M. Schwemmer, and K. F. Wong-Lin., “A Short Course in Mathematical Neuroscience(revised7/2015),” Ictp-saifr.org.[Online]. Available: https://www.ictp-saifr.org/wp-content/uploads/2015/05/MathNsci.pdf. [Accessed: 15-May-2023]
G. A. Silva, “The need for the emergence of mathematical neuroscience: beyond computation and simulation,” Front. Comput. Neurosci., vol. 5, p. 51, 2011
“The journal of Mathematical Neuroscience,” SpringerOpen. [Online]. Available: https://mathematical-neuroscience.springeropen.com/. [Accessed: 15-May-2023]
F. G. Ashby and Y.-W. Wang, “Computational cognitive neuroscience models of categorization,” in The Cambridge Handbook of Computational Cognitive Sciences, Cambridge University Press, 2023, pp. 400–425.
C. Gilmore and L. Cragg, “Teachers’ understanding of the role of executive functions in mathematics learning,” Mind Brain Educ., vol. 8, no. 3, pp. 132–136, 2014.
W. Yao, Y. Li, Z. Ou, M. Sun, Q. Ma, and G. Ding, “Dynamic analysis of neural signal based on Hodgkin–Huxley model,” Math. Methods Appl. Sci., vol. 46, no. 4, pp. 4676–4687, 2023.
A. G. Giannari and A. Astolfi, “Model design for networks of heterogeneous Hodgkin–Huxley neurons,” Neurocomputing, vol. 496, pp. 147–157, 2022
R. Rojas, “Das Modell von McCulloch und Pitts,” in Theorie der neuronalen Netze, Berlin, Heidelberg: Springer Berlin Heidelberg, 1993, pp. 29–50
S. Kumar et al., “Novel method for safeguarding personal health record in cloud connection using deep learning models,” Comput. Intell. Neurosci., vol. 2022, p. 3564436, 2022.
S. Kumar, P. K. Srivastava, G. K. Srivastava, P. Singhal, D. Singh, and D. Goyal, “Chaos based image encryption security in cloud computing,” J. Discrete Math. Sci. Cryptogr., vol. 25, no. 4, pp. 1041–1051, 2022.
S. Kumar et al., “Protecting location privacy in cloud services,” J. Discrete Math. Sci. Cryptogr., vol. 25, no. 4, pp. 1053–1062, 2022.
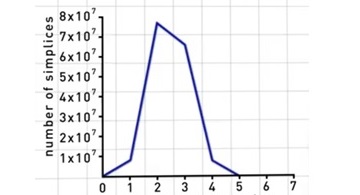
Downloads
Published
How to Cite
CITATION COUNT
Issue
Section
License
Copyright (c) 2023 Trisha Srivastava, Dr. Ambrish Pandey

This work is licensed under a Creative Commons Attribution 4.0 International License.